Fitzpatrick, M. C., Gotelli, N. J., & Ellison, A. M. (2013). MaxEnt versus MaxLike: empirical comparisons with ant species distributions. Ecosphere, 4(5), art55–15. http://doi.org/10.1890/ES13-00066.1
The output indices of MaxEnt are not truly direct estimators of the probability of species occurrence, but rather “ill-defined suitability [indices] (Royle et al 2012). In response to this, MaxLike, a formal likelihood model that generates ‘true’ occurrence probabilities using presence-only data, has been proposed as an alternative and shown to generate range maps that more closely match those of logistic regression models. However, it is unclear whether it can be generalized to SDMs to the extent that MaxEnt has been because the only comparison case so far used a larger sample size than is most often available, included the full geographic range of the species (and most studies cannot), and modified MaxEnt’s default settings, which may have reduced MaxEnt’s performance. As a test of generalization, Fitzpatrick et al compared MaxEnt andMaxLike models for six species of ants in New England, comparing outputs with goodness of fit, predictive accuracy measures, and comparison to expert opinion.
The authors began with 19 environmental variables, but then reduced to three: Annual Temperature and Rainfall, and Elevation. In doing so, they may have biased their study, as MaxEnt may be more robust to having multiple correlated or irrelevant variables than MaxLike. They then created 50 MaxEnt and 50 MaxLike models. The default settings were chosen for the MaxEnt models, and created a sampling bias surface based on the full dataset of ant occurrence records for 132 species was used to correct bias. Interestingly, a bias surface of all 132 species decreased MaxEnt performance, perhaps becuase the bias of the six focal species did not match that of the full dataset. Indeed, when the model was fit with a bias surface of only six species, it was a marginal improvement over the non-bias corrected models.
Goodness of fit was calculated with AIC and normalized Akaike model selection weights. Because AUC is especially problematic with presence-only data (WHY), two other measures of accuracy, minimum predicted area and mean predicted probability, were also examined. The authors have been working in this ant system for decades, so they were also able to compare models of distribution to expert knowledge and experience, a rarity, in my opinion, as many modelers are using data from systems they are unfamiliar with.
MaxLike models were better supported by the data, but model evaluation by AUC was inconclusive, although generally bias correction decreased the AUC of MaxEnt. In general, MaxEnt underestimated the probability of occurrence in areas where there were presence records, but over-estimated in unsampled areas. This is most likely due to the fact that MaxEnt assumes a mean probability of 0.5 for presence data, reducing the range of occurrence probabilities. Even with small data sets (to a minimum of five presence points) MaxLike more accurately predicted occurrence probabilities. Notably, because the authors created 50 models of each, a measure of uncertainty is available. In general, MaxLike had greater uncertainty, especially in areas with few presence points, which seems to be a fair and accurate conclusion to be drawn that machine learning methods often omit. MaxLike is able to perform better than MaxEnt on sparse data sets, even when MaxEnt is fit using default settings, and has the additional benefit of portraying uncertainty more accurately.
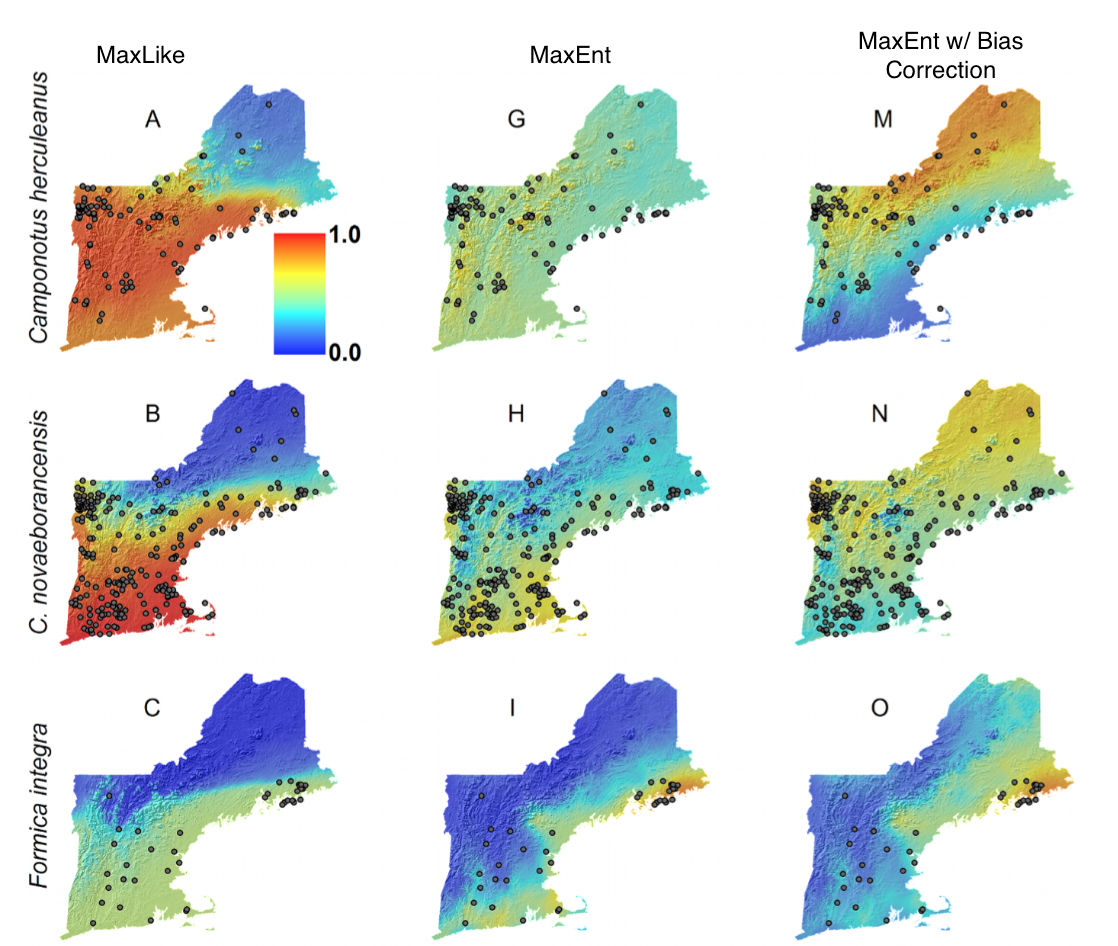