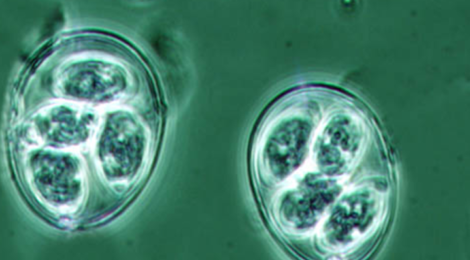
Predicting cryptic links in host-parasite networks
Host-parasite networks are built from a vast number of interactions, which may change seasonally or based on host densities. This combination of complexity and uncertainty means that the majority of host-parasite associations are poorly understood or not known at all. The effects of such “cryptic associations” between hosts and parasites are unknown. To address this knowledge gap, UGA ecologists developed a new approach to identifying cryptic associations, and applied their theory to empirical data to test the ability of a simple algorithm to predict interactions. The algorithm uses host and parasite trait data to train models that predict the probability of host-parasite interaction. They tested the accuracy of this approach using simulated networks with different properties, demonstrating high accuracy and robustness. They then applied this algorithm to data on a small mammal host-parasite network and estimated model accuracy. This also let them identify which host and parasite traits were important for predictions, and quantify how network structure changed when cryptic associations were added.
Dallas, T.A., A.W. Park, & J.M. Drake. 2017. Predicting cryptic links in host-parasite networks. PLoS Computational Biology 13(5):e1005557. [online] [data & code]