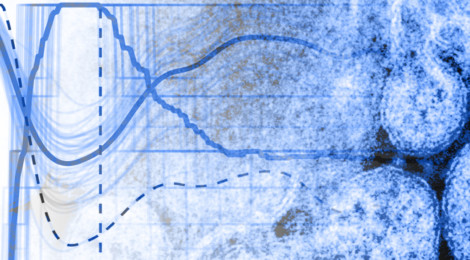
A data-driven semi-parametric model of SARS-CoV-2 transmission in the United States
As infectious disease modelers, one of the greatest challenges we face is in accurately reflecting the complexities of transmission, particularly human behavior. Important factors we must consider, like adherence to public health measures and variations in social distancing, are difficult or even impossible to measure directly. We are then led to one fundamental question: how do we quantify the unquantifiable?
In this paper, we offer an attempt to answer this question through the use of a semi-parametric model utilizing a stochastic SEIR framework. We focused on identifying the factors influencing transmission dynamics during the first year of the pandemic in the United States, including human mobility data derived from cellular devices and other observable data. To address the question of accounting for the unknowns, we used a novel latent process (represented by a time-dependent spline function) for capturing factors like the environmental and behavioral changes in the human population discussed above. This allowed our model to adapt to changing dynamics beyond what can be observed by inferring the impact of these unmeasurable factors based on the discrepancies between observed data and expected outcomes.
This model is one of many innovative new approaches that have emerged since the start of the pandemic. Our hope is that it will allow for a flexible approach to modeling disease transmission that, while imperfect, can effectively account for the complexities of human behavior and the effect that these behaviors and disease transmission have on each other.
To learn more, find this paper at PLOS Computational Biology.
Corresponding Author: John Drake, jdrake@uga.edu