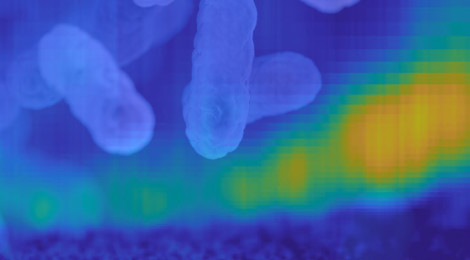
Anticipating epidemic transitions in metapopulations with multivariate spectral similarity
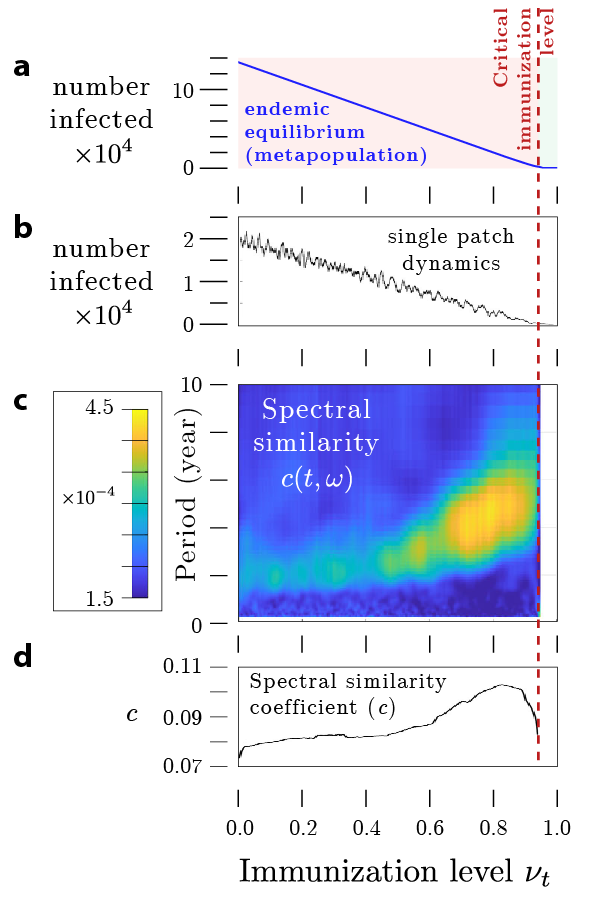
The control and prediction of emerging pathogens are major challenges for the health and safety of the public, as they are among the most unpredictable threats to global health and security. Disease outbreaks caused by viruses such as SARS-CoV-2 (COVID-19) and endemic illnesses such as tuberculosis, malaria, and dengue continue to pose significant health and economic burdens on much of the world population. It is clear that novel methods are needed to anticipate, understand, and intervene in the emergence and elimination of these myriad diseases.
In this paper, published in Nonlinear Dynamics, we propose a multivariate wavelet analysis method for anticipating tipping points in the dynamics of infectious diseases in a given population. It involves monitoring the similarity between the time series data obtained from the dynamic disease model at different locations to reveal information about the underlying system dynamics and its proximity to a critical point. Using this method, we analyze the temporal evolution of spectral similarity in multivariate epidemiological time series to reveal significant changes in the pattern of similarity cross-spectra.
We validated this method by analyzing a standard SIR metapopulation model and historical case reports of whooping cough in the USA. This approach, in conjunction with other methods, contributes to a better understanding of the emergence and elimination of diseases by measuring interrelation and similarity between system components and the frequency at which the interrelation dominates.
Corresponding Author: Amin Ghadami, ghadami@usc.edu