Fithian, W., Elith, J., Hastie, T., Keith, D. A. (2015), Bias correction in species distribution models: pooling survey and collection data for multiple species. Methods in Ecology and Evolution, 6: 424–438. doi: 10.1111/2041-210X.12242
Presence only records are common for rare species, but are often biased due to a haphazard collection schemes. The authors propose a correction for this bias by using presence – absence data with similar geographic sampling biases from other species.
Most popular presence only models are motivated by an inhomogeneous Poisson process (IPP). The IPP for a single species presence only data can be extended to adjust for sampling bias by incorporating presence – absence data from multiple species into a single joint probabilistic model to estimate and adjust for bias. The authors evaluate their model using both presence – only an presence – absence data for a set of Eucalypt species from south–eastern Australia (R package multi–speciesPP). Presence – only point processes can be thought of as a thinned presence – absence point process. How and where the thinning occurs is biased by opportunistic presence only sampling. See figure 1 for visual explanation. This means, at best, presence only IPP estimate relative intensities not probabilities of occurrence. This is due to the identifiability issue of parameters in the thinned intensity function.
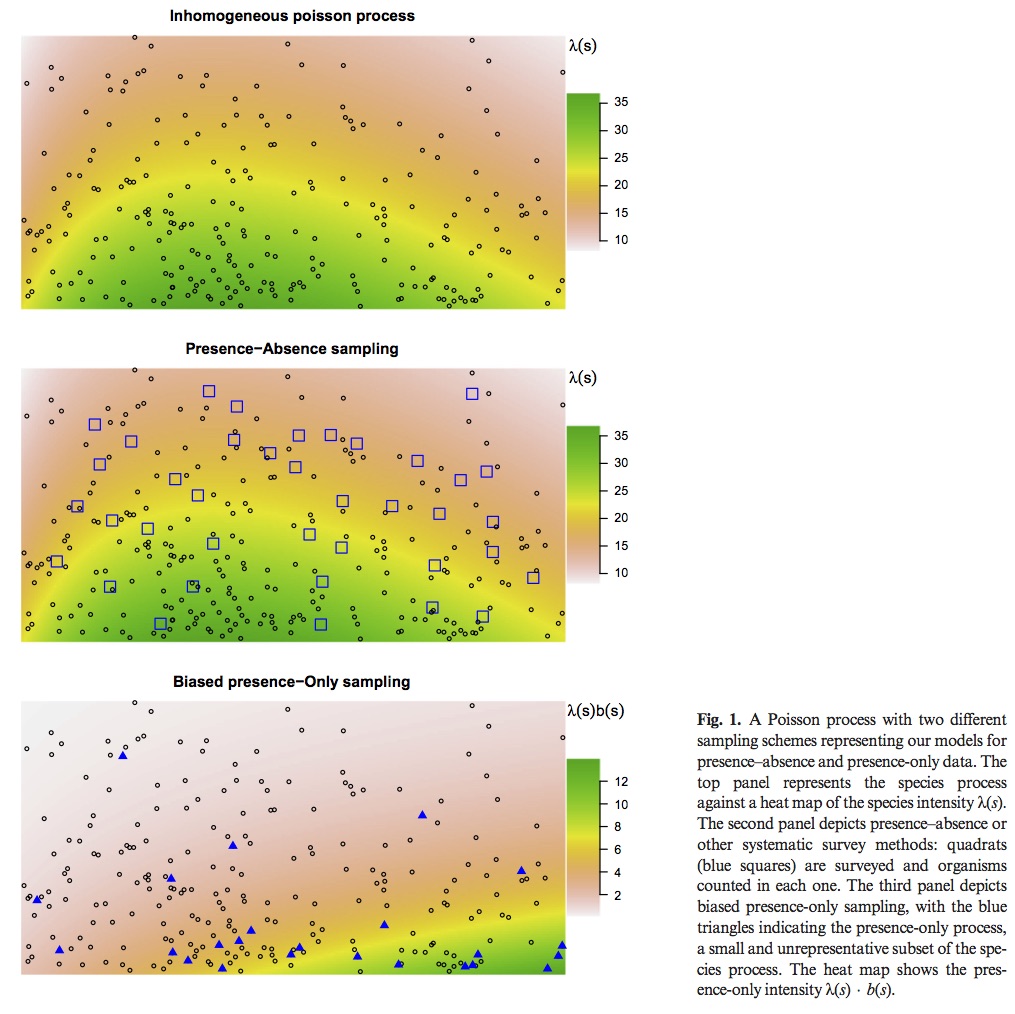
Previous attempts to correct for this bias have included factors that lead to sampling bias such as distance from roads and population centers. However, these corrections only work if they do not correlate with environmental variables. In Australia large populations are clustered along the East Coast, but important climatic variables are also correlated with distance from the same coast.
The authors propose using a joint log linear IPP model for multi-species data, a subset of which are presence – absence data. The point process and send point process are both assumed to be independent across species with a log linear intensity and bias, however bias intercept (delta) is not allowed to vary across species. This restriction assumes that bias is proportional across species which allows the authors to pool the information into a single estimate – deriving the bias of presence only data from presence absence data.
Testing the method:
The Eucalypt data set consists of 36 species at 32,612 sites with an average of 547 presences per species. However, this range is variable 4 species have fewer than 20 observations and 8 having more than 1000. The presence only data consists of 764 observations supplemented with 40,000 background points. The authors evaluated their methods by assessing the assumption of proportional sampling bias, and the impact of pooling multiple species on predictions.
The proportional bias assumption was found to be appropriate in some species, and inappropriate in others. Pooled data had the greatest impact on model performance when the presence absence data for species of particular interest were either scarce or nonexistent. The authors acknowledge the proposed method has many shortcomings, but point out that it performs better than models with no sampling bias correction.